Time evolution of probabilities
The discret version of pdf allows one to understand the dynamics in time.
If
is the probability of
at time
then
is the probability density of
at time
.
Let see the following example..
Example :
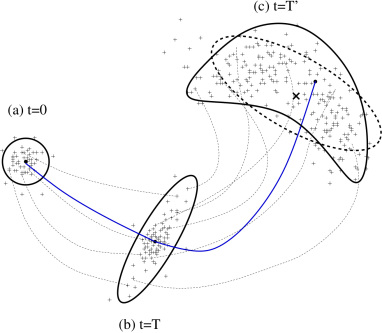
or this one..
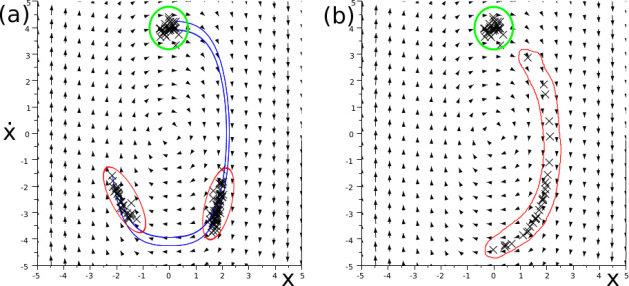
(a) At the linear approximation, the initial circle is transformed into ellipses following
a where its covariance matrix is
is
is a Gaussian random vector of covariance matrix
.
(b) Then, after a larger time of integration, the ensemble transformed by the flow is no more Gaussian, and this marks the first stage of nonlinear behaviour.